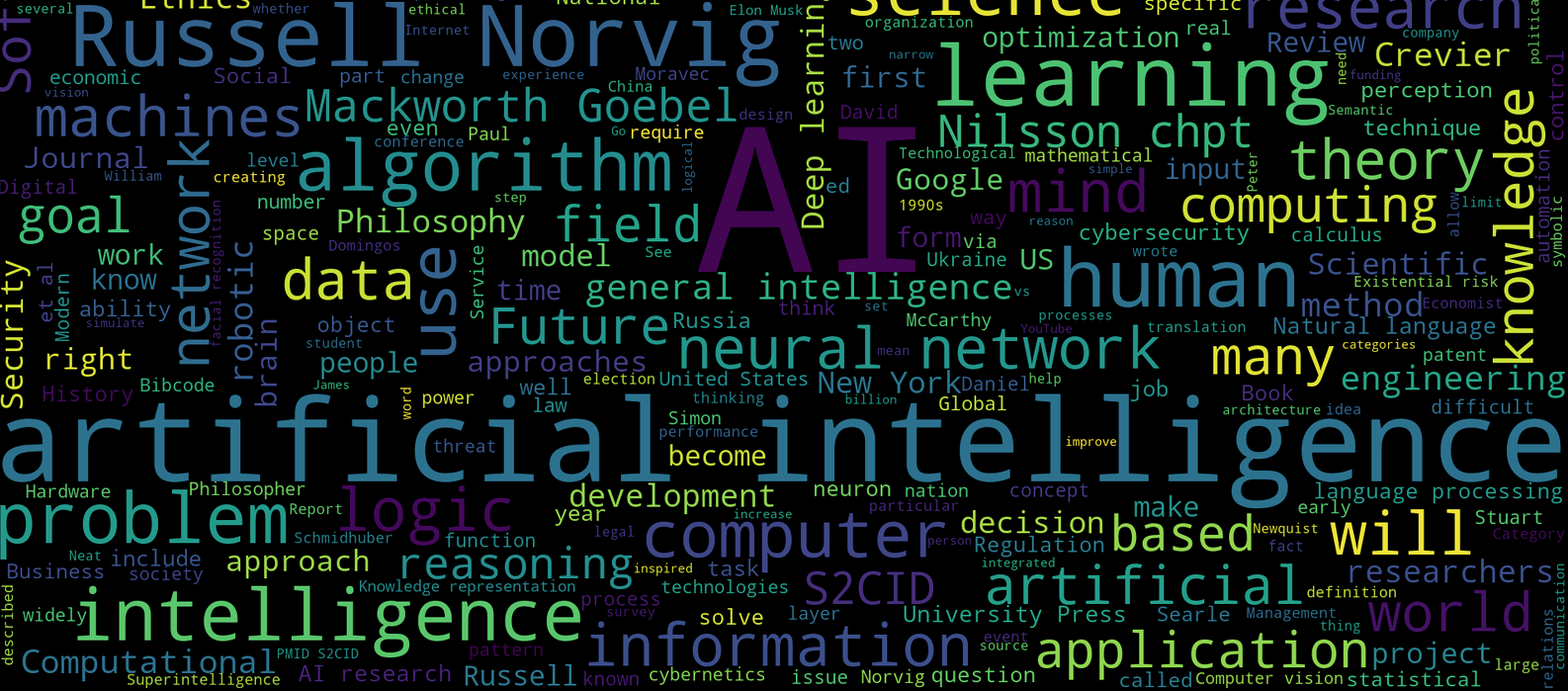
In a recent blog post, Vasant Dhar had some interesting remarks about the possible role of AI in Fed policy:
Might AI help us better understand the data and design better economic policy interventions?
Last week I had lunch with some central bankers who wanted to talk to me about AI. They were interested in discussing how they should think about the risks and opportunities associated with the increasing presence of AI in financial markets. I asked them the same question I had asked Paul: could AI do the work of the central bank? Over the course of the meal, they realized that an AI would indeed have a lot to offer in terms of connecting the dots better than humans can. The dots would include historic data on all past central bank monetary policy decisions, past communication and forecasts, all past and contemporary data available at the time, and all literature published about the subject over time. In principle, we felt that the AI might do a better job of modeling the economy. At the very least, its models should be compared to the ones currently in use.
But we’d still need humans to blame, they concluded. After all, you can’t get mad at an AI if it’s wrong.
I can certainly see the attraction of using AI in the formulation of monetary policy. But on balance, I’d still prefer to rely on a market approach to policy.
Would an AI be able to forecast better than an NGDP futures market? That’s hard to say. An AI forecast might incorporate market forecasts, and also other factors missed by market participants. In that case, you could think of AI forecasts as man/machine hybrids. In the early days of computer chess, play that combined the insights of both grandmasters and computer programs was better than either man or machine working in isolation.
But if AIs are truly superior to the market in certain respects, then we can expect market participants to use AI when engaged in the trading of financial assets. If so, then the insights of various AIs will become incorporated into market prices.
From this perspective, the addition of AI is not a qualitative change in markets or forecasting. Rather the AI revolution will add lots of really smart “entities” to the market, making it even more efficient than before. This may be important, but it’s still more of a quantitative than qualitative change. Even if some AIs are smarter than any individual, including Jay Powell, no individual AI will be smarter than a market that includes lots of smart humans and lots more even smarter AIs. The wisdom of crowds still holds.
A potentially more promising use of AIs would be to come up with the appropriate target of Fed policy (say inflation vs. NGDP growth, or levels vs. growth rates.) Those questions cannot be answered by market forecasts, as there is no point at which future data clearly resolves the question of who was right or wrong. Of course this is true of a wide range of public policy questions. Thus we could envision asking AIs whether the death penalty increases or reduces the aggregate utility of society. If this thought makes you queasy, you can take some comfort in the fact that AIs are currently very far from being able to answer that sort of question, and it’s not clear they will ever be able to do so.
PS. David Beckworth asked an AI what it thought about using AIs in monetary policy.
READER COMMENTS
Kenneth Duda
May 8 2024 at 6:35pm
Scott,
The idea of using AI to solve some hard problem that people aren’t very good at is as old as the field of AI itself. In 1993, the professors in my AI class told us how the pentagon kept trying to get AI researchers to create systems for solving military logistics. They’d say, “great! Please introduce us to the human expert who thoroughly understands how to solve this.” And the military was like, “umm…..”
There is no AI breakthrough on the horizon that can tackle something like monetary policy. We only have one recent breakthrough that can actually solve problems better than people, which is google’s AlphaZero system. It does amazingly well when the problem is space is small, good outcomes are easy to evaluate, and the behavior of the system is well enough understood at a micro-level that AlphaZero can simulate its way through billions of options. AlphaZero far exceeds human performance at chess, go, shogi, and protein folding. But monetary policy, or even driving yourself to work, are outside of the space where AlphaZero can succeed. And don’t even get me started on the idea of using LLM’s to solve problems like this. LLMs are great at one thing, and only one thing, which is language. Anything else they do is a lucky accident achieved by parroting human experts so effectively that it appears the LLM is solving a problem, when really, it’s just … well, parroting.
-Ken
Scott Sumner
May 9 2024 at 11:26am
Thanks. That was also my impression, but I’m glad to see it confirmed by someone who knows more about it than I do.
JoeF
May 9 2024 at 2:02pm
LLMs (very large deep learning models) are not limited to language at all. For just one example, a number of groups have recently used “AI” (based on LLMs) for weather forecasting. These models already show more skill than the numerical (physics-based) models that have been used for decades. The AI forecasting models work well because they have access to many decades of weather data that they can use a training. Some have speculated that the AI models may not do well when confronted with unprecedented weather conditions, whereas traditional numerical models (based on physics) would be fine. But the AI models are trained on weather from all over the globe over many decades, so this seems unlikely. The AI models also have another advantage over traditional rules-based models. Namely, you can add any input data (just as a hypothetical, dust concentrations in the atmosphere) and if it helps the prediction, the model will use it. No one has to “figure out” how to use the new data. I don’t know (at all) whether LLMs would be applicable to Fed policy.
Jeff
May 8 2024 at 11:22pm
This thought experiment actually crystallizes why markets and “wisdom of crowds” are not a solution for monetary policy. Imagine an AI that is instructed to do whatever it takes with its policy lever (say the interest rate or the monetary base, it doesn’t much matter) to maintain NGDP on a 4% glide path using an NGDP futures market as input. The market starts out with people making bets based on real observations and estimates, and the AI gradually “learns” a response function that tells it how much it needs to move the policy lever in response to the dynamics it sees in the futures market. But, over time, the nature of market changes, because the policy lever is powerful, and the AI is fairly capable, and since the AI is supposed to do whatever it takes to stay on target, it becomes pointless to make any significant bet against it. In this regime, forecasts rarely deviate much from the 4% goal, and the target seems almost to achieve itself, as if by magic. But what also happens at this point is that the response function the AI is using to train itself becomes meaningless. The market is less and less based on real observables and more and more a simple vote of confidence in the ability of the AI to handle whatever is thrown at it. Conversely, if the market begins to indicate any substantial deviation from the target in this regime, it’s not a simple signal that “course correction is needed” but rather a signal that “the situation appears unrecoverable”. That is, market participants are saying that observables suggest a state of affairs so far outside the domain of competence of the AI that total failure may be inevitable. So, it may not fail very often, but when it does so, it does so spectacularly.
Scott Sumner
May 9 2024 at 11:31am
You are describing the “circularity problem”, but this does not apply to the “guardrails” approach that I have advocated.
A better way to think about NGDP futures targeting is that the market isn’t so much forecasting NGDP as it is forecasting the instrument setting required to reach on-target NGDP. So the NGDP futures price never moves.
My new book has a chapter discussing the idea if you are interested.
spencer
May 9 2024 at 11:55am
The banks aren’t reserve constrained. Neither is the E-$ market.
The NBFIs are the DFI’s customers:
“The spread widened because liquidity for non-banks became scarcer relative to that for banks due to quarter-end corporate tax payments”
Balance Sheet Normalization: Monitoring Quantities and Prices | St. Louis Fed (stlouisfed.org)
MarkLouis
May 13 2024 at 7:52pm
If AI can forecast better than markets, it will quickly be incorporated into markets. It probably already is. Remember, large hedge funds have immense resources and little incentive to talk about what they’ve discovered.
Comments are closed.